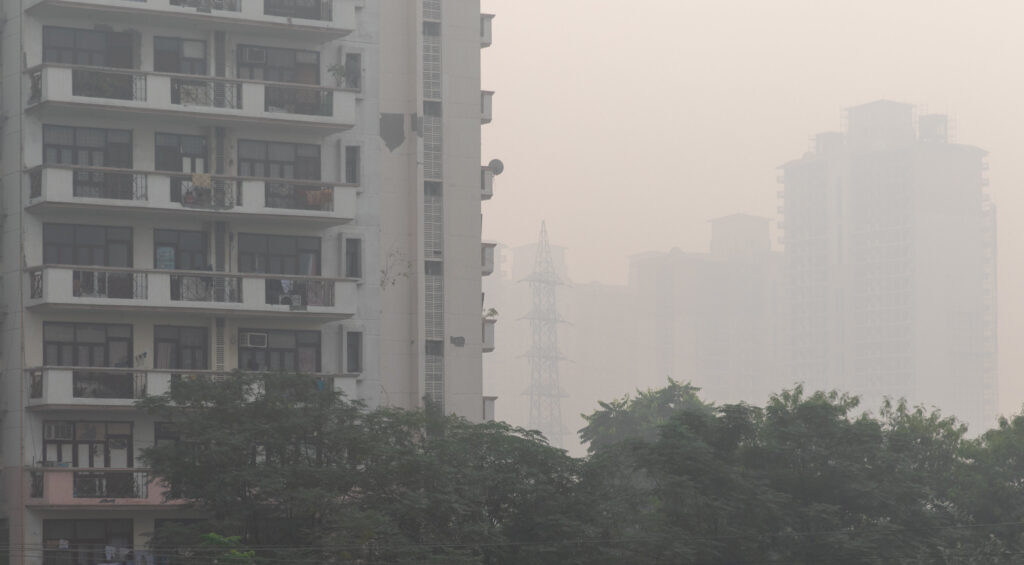
Poor air quality is a major global problem. According to the World Health Organization, exposure to air pollution is linked to the premature deaths of an estimated seven million people every year. In fact, 9 out of 10 people breathe air that contains more pollutants than what the WHO considers safe. Air pollution is the fourth largest threat to human health, trailing only high blood pressure, dietary risks, and smoking.
But predicting pollution levels at a given place and time remains challenging. According to a new study recently published in the journal Science of the Total Environment, scientists are turning to deep learning to improve air quality estimates.
According to researchers, satellite observations and ground observations both measure air pollution, but both have major limitations. For example, satellites may collect data at the same time and at the same location each day, but they miss how emissions may vary throughout the day. Ground-based observations from weather stations do continuously collect data, but they only do so in a limited number of locations.
As a result, scientists have turned to deep learning – a type of machine learning – to analyze the relationship between satellite and ground-based observations of nitrogen dioxide around Los Angeles. Nitrogen dioxide is associated with emissions from traffic and power plants. The researchers were able to rely on the learned relationship to take daily satellite observations and create hourly estimates of atmospheric nitrogen dioxide levels in approximately three mile grids.
According to the research team, this study could be repeated for other greenhouse gases, and applied to different cities and regions – or even whole continents.
**********
Web Links
Scientists turn to deep learning to improve air quality forecasts
Photo, posted November 4, 2019, courtesy of Ninara via Flickr.
Earth Wise is a production of WAMC Northeast Public Radio.
Leave a Reply